The Finnish Land Parcel Identification System (FLPIS) supports the calculation of national and European Union (EU) farm subsidies paid by the Finnish Food Authority. In 2023, there were more than 50,000 subsidy beneficiaries. The amount of aid depends on the area under cultivation, so the FLPIS is a hub of geometrical farmland data.
Satellite imagery plays an increasingly crucial role in agricultural land monitoring in the context of the EU’s Common Agricultural Policy. Datasets generated by satellites are complemented with information collected from farmers and rural affairs authorities though online surveys. Such surveys seek to establish, for example, which areas farmers intend to cultivate and for what areas they are claiming aid.
Enabling on-time, efficient payments
It is vital that farm subsidies are paid to Finnish farmers on time and in the right amounts. This requires reliable databases and up-to-date registration data. FLPIS is a critical system in this respect.
Through CGI’s managed services partnership with the Finnish Food Authority, our team performs development and maintenance of the FLPIS to enable on-time, efficient payments to farmers. This includes the use of satellite imagery, AI, and cloud computing technologies to improve the performance of FLPIS through advanced farmland monitoring and data processing.
Sophisticated interpretation of satellite data is a critical part of modern farmland analysis. It requires understanding the effects of natural resources on different stages of the growing season, methods of deciphering satellite images based on AI, and ways to enable the calculation of large volumes of data through cloud computing. Satellite monitoring is a key area of expertise for CGI.
The FLPIS uses machine learning to analyze about 1 million land parcels throughout the growing season, representing Sentinel-2 satellite imagery data for more than 100 million square kilometers (km2).
With the help of the system developed by CGI, monitoring is comprehensive, and at the same time cost savings are achieved and valuable information is created, for example, on the calculation of the condition of agricultural land, yield potential and fertilization needs.
Project Manager, Finnish Food Authority
Reducing field visits and improving program equality
Monitoring farmland parcels formerly was a manual effort. Now, FLPIS automates monitoring for nearly 100% of the surface area, or 2.2 million hectares.
The system uses machine learning to analyze vast data from Sentinel-2 satellites, along with field parcel data, to aid in determining subsidies and monitoring farmland conditions. An XGBoost (or Extreme Gradient Boosting) forest model helps identify what’s grown on which field, for example.
“The main benefits of the system will be reduced off-road visits and improved equality. Every parcel will be included in the monitoring, not just those selected for field visits, says Åke Möller.
* For subtitles, please use YouTube’s closed captions (CC) and auto-translate features. Learn more.
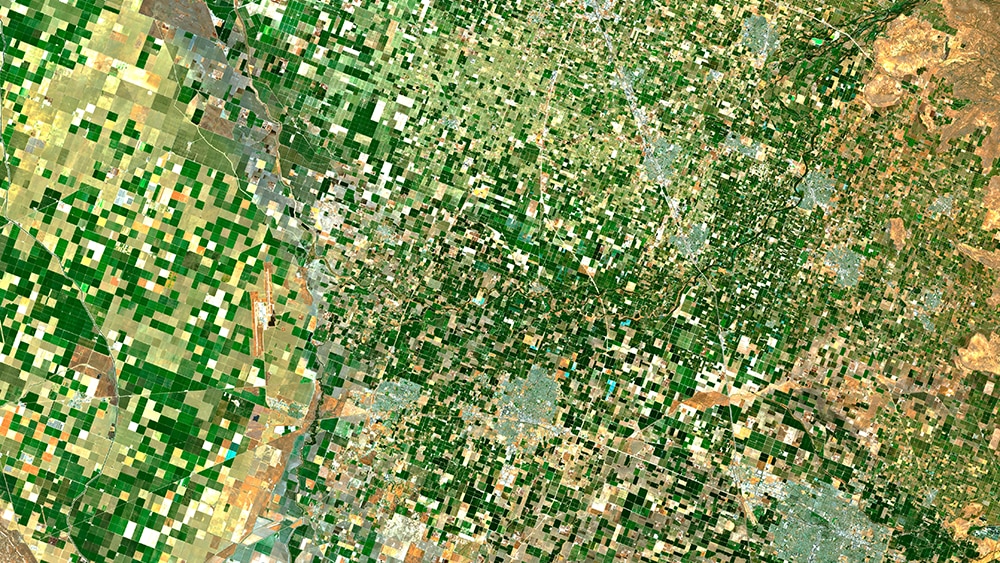